The use of language models (LMs) has exploded in recent years, and ChatGPT is just the tip of the iceberg. ChatGPT has been used to write code, recipes, or even sonnets and poems. All noble purposes, but there is also a huge scientific literature, so why not exploit this vast amount of textual data?
Microsoft recently unveiled BioGPT which does exactly that. the new model has achieved state-of-the-art in several tasks. Let’s find out together.
Meanwhile, why is it important? Thousands and thousands of scientific publications come out every year, and it is difficult to be able to keep up with the growing literature. On the other hand, the scientific literature is essential to be able to develop new drugs, establish new trials, develop new algorithms, or understand the mechanisms of disease.
In fact, NLP pipelines can be used to be able to extract information from large amounts of scientific articles (names and entities, relationships, classification, and so on). On the other hand, LMs models generally have poor performance in the biomedical area (poor ability to generalize in such a domain). for this reason, researchers, prefer to train models directly on scientific literature articles.
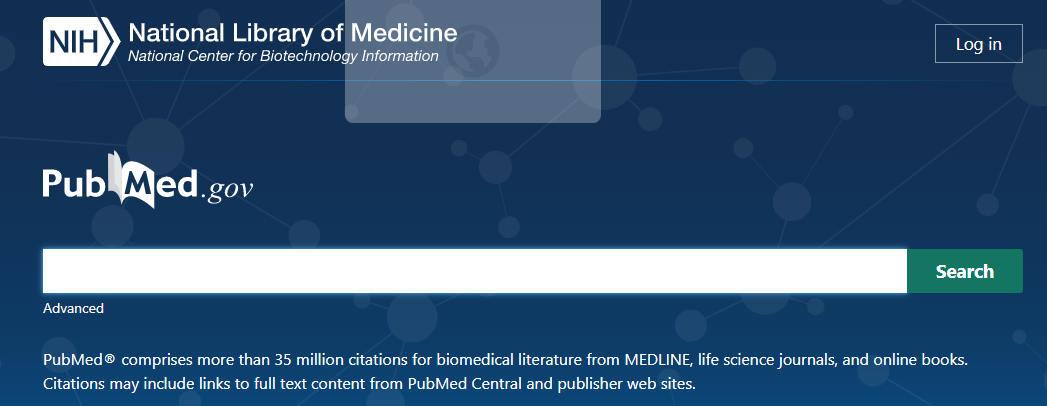
In general, Pubmed (the main repository of scientific articles) contains 30M articles. So there are enough articles to be able to train a model and then use this pre-trained model for follow-up tasks.
Generally, two types of pre-trained models were used:
- BERT-like models, trained using masked languaging modeling. Here the task is given a sequence of tokens (subwords) some are masked, and the model using the remaining tokens (context) should predict which tokens are masked.
- GPT like, trained using auto-regressive language modeling. The model learns to predict the next word in a sequence, knowing which words are before in the sequence.
BERT has been used extensively and in fact, there are several alternatives dedicated to the biomedical world: BioBERT, PubMedBERT, and so on. These models have shown superior capabilities in understanding tasks in compared to other models. On the other hand, GPT-like models are superior in generative tasks and have been little explored in the biomedical field.
So in summary, the authors in this paper used GPT-like architecture:
we propose BioGPT, a domain-specific generative pre-trained Transformer language model for biomedical text generation and mining. BioGPT follows the Transformer language model backbone, and is pre-trained on 15M PubMed abstracts from scratch. We apply BioGPT to six biomedical NLP tasks: end-to-end relation extraction on BC5CDR [13], KD-DTI [14] and DDI [15], question answering on PubMedQA [16], document classification on HoC [17], and text generation. (original article)
The authors tested (and compared with previous methods) BioGPT on three main tasks:
- Relation extraction. The purpose is the joint extraction of both entities and their relationships (e.g., drugs, diseases, proteins, and how they interact).
- Question answering. In this task, the model must provide an appropriate answer according to the context (reading comprehension).
- Document classifcation. The model must classify (predict) a document with a label (or more than one label).
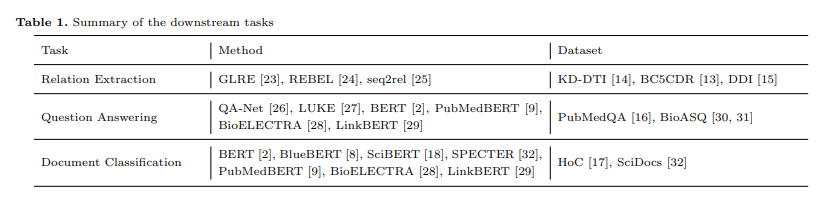
As the authors point out when training a model from scratch it is important to make sure that the dataset is from the domain, of quality, and in the right amount. In this case, they used 15 M abstracts. In addition, the vocabulary must also be appropriate for the domain: here the vocabulary is learned with byte pair encoding (BPE). Next, the architecture must be chosen, and the authors chose GPT-2.
The authors, specifically engined the datasets to create training prompts (in which they provided the prompt and target). This was to enable better training specific to the biomedical domain.
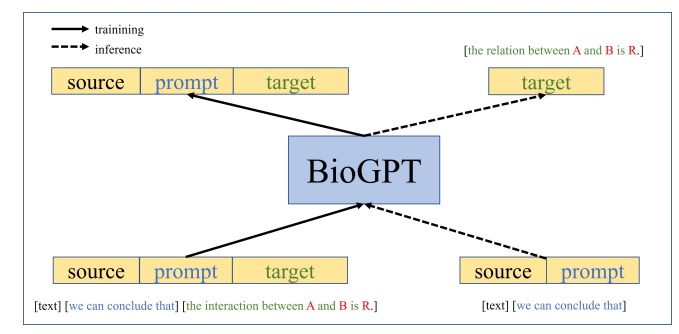
The authors evaluated the model on end-to-end relationship extraction with a model called REBEL (based on BART, a variant of BERT) and which was recently published. They also used as a baseline the GPT-2 model, which has not been trained specifically for the medical domain. The table shows that BioGPT achieves state-of-the-art in a chemical dataset:
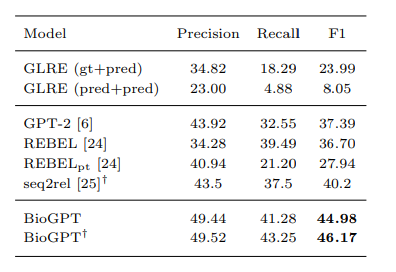
“Results on BC5CDR chemical-disease-relation extraction task. ’gt+pred’ means using ground truth NER information for training and using open-source NER tool to annotate NER for inference. ’pred+pred’ means using open-source NER tool for both training and inference. ’†’ means training on training and validation set. M”. image source: preprint
drug-drug interaction is another type of interaction that is very useful in research. In fact, interactions between two different drugs are among the leading causes of adverse effects during treatment, so predicting potential interactions going forward is an asset for clinicians:
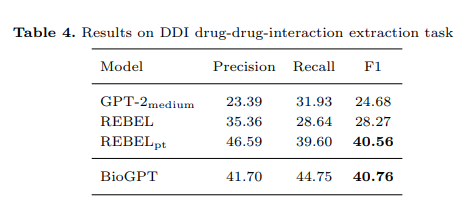
They also used a drug-target interaction dataset. The result is very interesting because predicting drug-target interaction can be useful for developing new drugs.
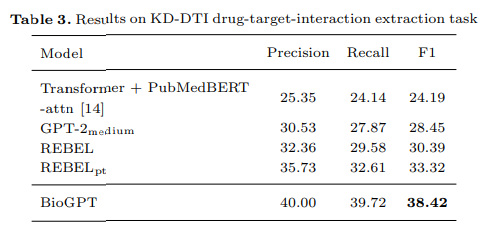
In this task, too, the model achieved state-of-the-art status:
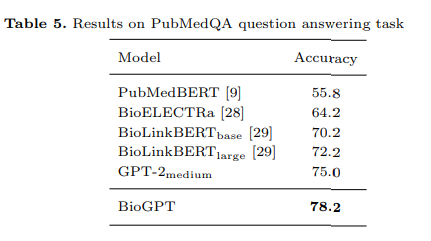
And even in document classification, BioGPT outperformed previous models
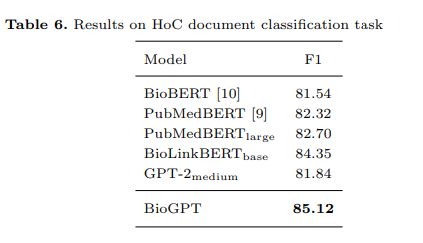
As said above, GPT has the generative capability: “ they can continue to generate text that are syntactically correct and semantically smooth conditioning on the given text”. The authors decided to evaluate the ability of the model in generating biomedical synthetic text.
Specially, we extract all the entities within the triplets from the KD-DTI test set (i.e. drugs and targets). Then for each drug/target name, we provide it to the language model as the prefix and let the model generate text conditioned on it. We then investigate whether the generated text is meaningful and fluent. (original article)
The authors noted that the model was working well with known names as input while if you input an unknown name the model or it is copying from the article (something seen in the training set) or fails in generating an informative test.
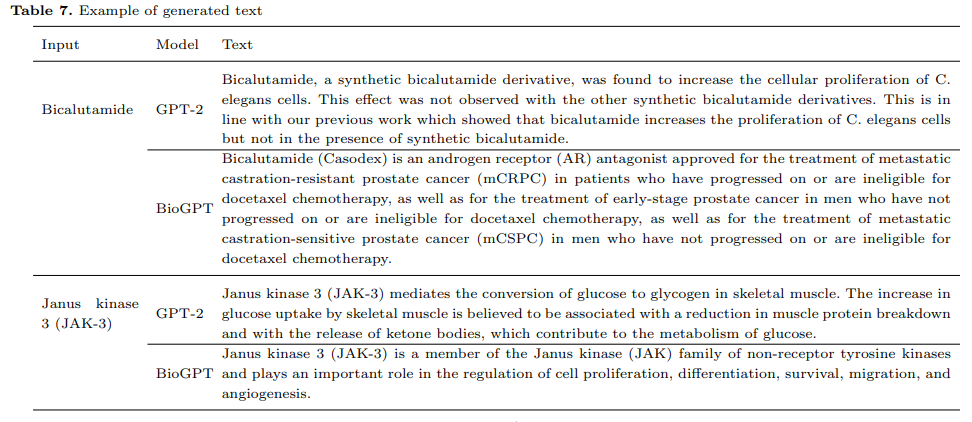
In conclusion:
BioGPT achieves SOTA results on three endto-end relation extraction tasks and one question answering task. It also demonstrates better biomedical text generation ability compared to GPT-2 on the text generation task. (original article)
For the future the authors would like to scale up with a bigger model and a bigger dataset:
For future work, we plan to train larger scale BioGPT on larger scale biomedical data and apply to more downstream tasks. (original article)
Microsoft is convinced it can be useful for helping biologists and scientists with scientific discoveries. The model can be useful in the future for the research of new drugs, being included in pipelines that are analyzing the scientific literature.
article: here, preprint: here, GitHub repository: here, HuggingFace page: here.
What do you think about it?
All Comments